An Overview of Experimental Design:
Experimental design, also known as the “design of experiment,” stands as a critical branch of applied statistics devoted to planning, executing, analyzing, and interpreting controlled tests. Its significance lies in its ability to assess the factors influencing a parameter or set of parameters, making it a robust tool for data collection and analysis in various experiments. In this article, we explore an overview of experimental design, including its principles, types, and applications across diverse fields.
What is Experimental Design?
Experimental design is a branch of applied statistics focused on planning, conducting, analyzing, and interpreting controlled tests or experiments. Its primary purpose is to systematically investigate and evaluate the factors influencing a specific parameter or set of parameters. By adhering to principles such as randomization, replication, and local control, experimental design ensures the generation of reliable and meaningful data. It is a crucial tool in scientific research, aiding in the formulation of hypotheses, testing of causal relationships, and reducing variability within treatment conditions. Experimental design is widely applied across various fields, providing a structured and efficient approach to answer research questions and advance knowledge.
Objectives of Experimental Design:
A well-crafted experimental design serves three primary objectives:
1. Causation: Experimental design aims to establish a causal relationship between independent and dependent factors. Causation refers to the ability of the experimenter to draw conclusions about the cause-and-effect connection between variables. By systematically manipulating the independent variable(s) and observing changes in the dependent variable(s), researchers can infer whether changes in the independent variable are responsible for the observed effects. This objective is critical for understanding the fundamental mechanisms and influences that drive specific phenomena, allowing researchers to make informed and valid deductions about the impact of certain factors.
2. Control: The objective of control in experimental design is to eliminate or minimize the influence of extraneous or confounding factors, ensuring that observed effects can be attributed to the manipulated independent variable(s) rather than external variables. Extraneous factors are variables that are not of primary interest but may inadvertently affect the dependent variable, leading to inaccurate conclusions. Through careful control, experimental design reduces the potential for alternative explanations, enhancing the internal validity of the study. This objective ensures that any observed changes can be confidently attributed to the experimental manipulation, strengthening the reliability of the experimental findings.
3. Variability: Experimental design seeks to reduce variability within treatment conditions, making it easier to detect and identify differences in treatment outcomes. Variability refers to the extent to which data points deviate from the mean or expected value. By minimizing variability within experimental conditions, researchers increase the precision of their measurements and enhance the ability to detect true differences or effects. This objective is crucial for obtaining accurate and consistent results, as reduced variability improves the reliability and replicability of the study. It also contributes to the overall robustness of the experimental design, allowing researchers to draw more dependable conclusions from their findings.
Basic Principles of Experimental Design:
Three fundamental principles underpin experimental design:
1. Randomization: Randomization is a fundamental principle in experimental design that involves the random assignment of treatments to different experimental groups. This process aims to eliminate bias and ensure that each experimental group is equally likely to receive any of the available treatments. By randomizing the assignment of treatments, researchers can create comparable groups, reducing the likelihood of systematic differences between them. This principle is crucial because it helps control for unknown or unmeasured variables, making the experimental groups more representative of the larger population. Randomization enhances the internal validity of the study by minimizing the impact of confounding variables, allowing researchers to confidently attribute observed effects to the manipulated independent variable.
2. Principle of Replication: The Principle of Replication involves repeating the experiment with multiple instances or subjects to increase the accuracy and reliability of the results. Replication contributes to the precision of the study by providing a larger sample size and reducing the impact of random variability. When an experiment is replicated, researchers can assess the consistency of the observed effects across different instances, enhancing the generalizability of their findings. Replication also allows for the estimation of the variability within and between experimental conditions, providing a more robust basis for drawing conclusions about the relationships between variables. Ultimately, the Principle of Replication strengthens the scientific rigor of the experiment and enhances the confidence in the validity of the results.
3. Principle of Local Control: The Principle of Local Control addresses extraneous sources of variation that may not be eliminated by randomization and replication alone. Extraneous sources of variation refer to factors outside the experimental manipulation that could affect the dependent variable. Local control involves techniques such as balancing, blocking, and grouping of experimental units to bring these extraneous sources under regulation. Balancing ensures that key characteristics are evenly distributed among experimental groups, blocking involves grouping similar experimental units together, and grouping helps control for specific factors that may impact the experiment. By applying local control techniques, researchers enhance the efficiency and precision of the experimental design, minimizing experimental error and increasing the ability to detect true treatment effects.
Steps in Experimental Design:
The process of experimental design involves several key steps:
1. Identify the Problem: The first step in experimental design is to clearly define the research problem or question that the experiment aims to address. This involves precisely identifying the variables of interest and formulating a clear, specific, and testable research question. The researcher should have a comprehensive understanding of the context and purpose of the study, laying the foundation for subsequent steps in the experimental design process.
2. Establish Hypotheses: Once the research problem is identified, the next step is to establish hypotheses that offer potential solutions or explanations for the observed phenomena. Hypotheses typically consist of a null hypothesis (suggesting no effect or relationship) and an alternative hypothesis (indicating the expected effect or relationship). These hypotheses guide the experimental design by providing a framework for testing and drawing conclusions. Well-crafted hypotheses contribute to the clarity and direction of the research, guiding subsequent decisions in the design and execution of the experiment.
3. Design Experiment: With hypotheses in place, researchers proceed to plan the experiment to systematically test the formulated hypotheses. This step involves detailed planning of the experimental procedure, including the selection and manipulation of independent variables, measurement of dependent variables, and consideration of any control variables. Experimental design may also include decisions on the use of randomization, replication, and local control to enhance the validity and reliability of the study. The goal is to create a structured and controlled environment that allows for the systematic investigation of the research question.
4. Conduct Experiment: Once the experimental design is finalized, researchers move on to the execution of the designed experiment. This involves implementing the planned procedures, administering treatments, collecting data, and ensuring that the experiment is conducted under controlled conditions. Attention to detail during the execution phase is crucial to maintaining the internal validity of the study, ensuring that the observed effects can be confidently attributed to the manipulated independent variable rather than extraneous factors.
5. Analyze Data: After collecting the experimental data, the next step is a thorough analysis to extract meaningful insights. Statistical techniques are often employed to examine the relationships between variables, test hypotheses, and quantify the observed effects. The choice of analytical methods depends on the nature of the data and the experimental design. Rigorous data analysis is essential for drawing valid conclusions from the experiment, providing evidence for or against the formulated hypotheses.
6. State Conclusions: The final step in the experimental design process is to summarize and articulate the conclusions drawn from the experiment. Researchers interpret the results in the context of the hypotheses, discussing the implications of their findings and addressing the initial research problem. Clear communication of conclusions is vital for disseminating the results to the scientific community and contributing to the broader body of knowledge in the respective field.
Types of Experimental Designs:
Experimental designs fall into three main categories:
1. Quasi-experimental Design: Quasi-experimental designs are employed when true experimental conditions, such as random assignment or a control group, are difficult or impossible to implement. In this type of design, researchers still aim to examine the impact of interventions, but the study lacks certain elements of strict experimental control. Typically, quasi-experimental designs involve the use of pretests and posttests to measure the effects of an intervention. Unlike true experimental designs, quasi-experiments often lack random assignment of participants to different groups, making it challenging to establish a causal relationship conclusively. Examples of quasi-experimental designs include one-group pretest-posttest designs, non-equivalent control group designs, and interrupted time-series designs.
2. Pre-experimental Design: Pre-experimental designs are preliminary studies conducted before a full-scale true experiment. These designs help researchers assess the feasibility and potential impact of an intervention or manipulation, providing insights into whether a more extensive study is justified. Unlike true experiments, pre-experimental designs lack the rigorous control and randomization typically associated with experimental research. Common examples of pre-experimental designs include one-shot case studies, one-group pretest-posttest designs, and static-group comparisons. While pre-experimental designs may offer initial insights, their limitations, such as the absence of randomization and control groups, make them less conclusive compared to true experimental designs.
3. True Experimental Design: True experimental designs represent the gold standard in experimental research. These designs involve random subject selection, where participants are assigned randomly to experimental and control groups. The key characteristic of true experimental design is the manipulation of an independent variable to observe its impact on a dependent variable while controlling for extraneous factors through random assignment. Randomization enhances the internal validity of the study by minimizing biases and ensuring that any observed effects can be attributed to the experimental manipulation rather than other variables. True experimental designs include randomized controlled trials (RCTs), factorial designs, and repeated measures designs. These designs allow researchers to make strong causal inferences and establish the efficacy of interventions or manipulations.
Applications of Experimental Design:
Experimental design is a versatile and fundamental tool that finds applications across a wide range of fields, contributing to advancements in knowledge and innovation. Here’s an exploration of its applications in various domains:
1. Medicine: Experimental design plays a pivotal role in medical research, particularly in clinical trials and drug development. Randomized controlled trials (RCTs), a form of true experimental design, are commonly used to assess the effectiveness of new medications, treatments, or interventions. By randomly assigning participants to treatment and control groups, researchers can establish causal relationships and determine the efficacy of medical interventions. Experimental design is crucial in optimizing study protocols, ensuring reliable results, and advancing medical knowledge.
2. Engineering: In engineering, experimental design is applied to optimize processes, improve product design, and assess the impact of various factors on performance. For example, engineers may use factorial designs to investigate how different factors, such as material composition or manufacturing processes, influence the quality of a product. This systematic approach helps engineers identify optimal conditions, reduce variability, and enhance the efficiency of engineering processes.
3. Sciences: Experimental design is fundamental to scientific research across disciplines such as physics, chemistry, biology, and environmental science. Scientists use experimental designs to investigate hypotheses, control variables, and draw valid conclusions about natural phenomena. Whether studying the behavior of subatomic particles, conducting biological experiments, or analyzing environmental factors, experimental design provides a structured and rigorous approach to scientific inquiry.
4. Surveys and Opinion Polls: In social sciences, experimental design is applied to surveys and opinion polls to understand human behavior, preferences, and attitudes. Researchers design experiments to test hypotheses about the impact of interventions or information on public opinion. By carefully planning and executing surveys with randomized control groups, researchers can draw more accurate conclusions about the causal effects of specific interventions or messaging strategies.
5. Computer Programming: Experimental design is also relevant in computer programming and software development. Developers may use factorial designs to assess the impact of different programming languages, algorithms, or user interface designs on software performance. By systematically varying factors and measuring outcomes, programmers can optimize code, enhance user experience, and improve the overall efficiency of software systems.
6. Agriculture: In agriculture, experimental design is applied to optimize crop yields, evaluate the effectiveness of fertilizers, and study the impact of various agricultural practices. For example, researchers may design experiments to assess the influence of irrigation methods, soil types, or pest control strategies on crop productivity. This structured approach helps farmers and agricultural scientists make informed decisions for sustainable and efficient farming practices.
7. Quality Control: Experimental design is extensively used in quality control processes across industries. By systematically testing and varying factors that may influence product quality, manufacturers can identify optimal conditions and minimize variability in production. This ensures that products meet quality standards and helps in continuous improvement processes.
In conclusion, experimental design stands as a cornerstone in scientific research, offering a systematic approach to planning, executing, and interpreting controlled tests. By adhering to principles and following a structured process, researchers can derive meaningful insights and draw reliable conclusions, making experimental design an indispensable tool in advancing knowledge across diverse disciplines.
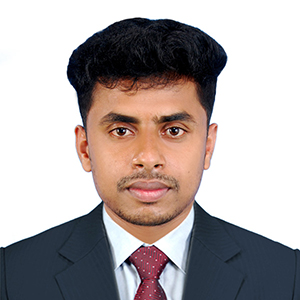
Assistant Teacher at Zinzira Pir Mohammad Pilot School and College