Data Analysis in Research
Data analysis is the process of cleaning, processing, and modifying raw data in order to obtain useful, pertinent information that supports making a decision. The data analysis process is basically done by three essential things — first data organization, second data reduction (facilitates the identification and connection of data by highlighting patterns and themes for easy identification), and last data analysis. The process can produce helpful insights and statistics, frequently presented in charts, graphics, tables, and graphs, which minimize the risks of making decisions. The purpose of it is to identify, transform, support decision-making, and bring a conclusion to research. In this article, we are going to know about the definition of data analysis, the types of data analysis, and the process of data analysis in research.
Definition of Data Analysis in Research:
Research data analysis is a process used by researchers for reducing data to a story and interpreting it to derive insights. The data analysis process helps in reducing a large chunk of data into smaller fragments, which makes sense (LeCompte and Schensul).
Data analysis is a messy, ambiguous, and time-consuming, but creative and fascinating process through which a mass of collected data is brought to order, structure, and meaning (Marshall and Rossman).
Data analysis is a process of inspecting, cleansing, transforming, and modeling data with the goal of discovering useful information, informing conclusions, and supporting decision-making (Wikipedia).
From the above definitions, we can say that the technique of data analysis frequently entails several steps, such as data collection, cleansing, and organization. Data analytics, another name for data analysis, is defined as the science of analyzing raw data in order to make acceptable statements about the data.
Types of Data Analysis:
Based on business and technology, there are various types of data analysis. Some of the major types of data analysis are briefly furnished below:
1. Text Analysis: Text analysis, which is sometimes known as “data mining,” makes use of databases and data mining technologies to find patterns in massive datasets. It converts unprocessed data into practical business knowledge. The easiest and most direct way to analyze data is probably through text analysis.
2. Statistical Analysis: Data collection, analysis, interpretation, presentation, and modeling are all included in statistical analysis. A set of data or a sample of data is analyzed. This form of analysis falls into two categories: Descriptive Analysis and Inferential Analysis.
- Descriptive Analysis: is used to describe the basic features of versatile types of data in research. It serves as the basis for all data understanding. It is currently the most straightforward and usual use of data in business. The descriptive analysis summarizes prior data, typically in the form of info-graphics, to provide an explanation for “what happened?”. It shows mean and deviation for continuous data whereas percentage and frequency for categorical data.
- Inferential Statistics: supports to development a decent understanding of the population data by analyzing the samples attained from it. It helps in making simplifications about the population by using various analytical tests and tools. In order to pick out random samples that will represent the population accurately many sampling techniques are used. Some of the essential approaches are simple random sampling, stratified sampling, cluster sampling, and systematic sampling techniques.
3. Diagnostic Analysis: Diagnostic analysis answers the question “Why did it happen?” by identifying the root cause, using the insights obtained from statistical analysis. Generating precise information is a crucial component of diagnostic analysis. When fresh issues come up, it’s probable that you’ve previously gathered some details about the issue. There is no need to repeat work because you already have the data available, and all issues are connected.
4. Predictive Analysis: “What is likely to happen?” is the question that predictive analysis intentions to explain. This kind of analytics utilizes statistical information to make predictions about future events. Its accuracy depends on how much information you have in-depth and how deeply you research it.
5. Prescriptive Analysis: this is the technically advanced data analysis, combining the facts from all prior analyses to specify the approach to be taken in a given scenario or choice. The latest technology and data management techniques are used in the prescriptive analysis. Companies must be sure they are prepared and willing to put forth the effort and resources because it is a significant organizational commitment. A prime example of prescriptive analytics is Artificial Intelligence (AI).
Process of Data Analysis:
According to John Tukey, “Procedures for analyzing data, techniques for interpreting the results of such procedures, ways of planning the gathering of data to make its analysis easier, more precise or more accurate, and all the machinery and results of (mathematical) statistics which apply to analyzing data.” Data Analysis consists of the following phases:
Data Requirements: You should first consider why you want to conduct this data analysis. You need to understand the objectives or goals of doing data analysis. The type of data analysis you intended to perform must be chosen. You should identify what to analyze and how to measure it at this phase, as well as why you are looking into the matter and the methods you will employ to conduct this Analysis.
Data Collection: You will have a clear understanding of what you need to measure and what your findings should be after gathering the requirements. It’s now time to gather your data based on the specifications. After gathering your data, keep in mind that it needs to be structured or processed for analysis. You must keep a diary detailing the sources and dates of data gathering as you gather information from various sources.
Data Processing: Data, when primarily attained, must be processed or structured for analysis. For instance, these may involve placing data into rows and columns in a table format (known as structured data) for further analysis, often through the use of spreadsheet or statistical software.
Data Cleaning: Now whatever the data has been collected, it may not be relevant to your analysis or usable, so it needs to be cleaned. The data that is collected could have duplicate records, blank spaces, or mistakes. Data should be error-free and thoroughly cleaned. Data cleaning must come before analysis since it will make the analysis’ result more likely to match expectations.
Exploratory Data Analysis: Once the datasets are cleaned, they can then be analyzed. As you operate data, you may figure out the exact information which you need, or you might need to accumulate more data. During this phase, you can use data analysis tools and software which will assist you to apprehend, interpret, and deriving conclusions based on the necessities.
Data Interpretation: After analyzing your data, it’s finally time to understand your results. You can decide on the way to express or communicate your data analysis either you can use simple words or maybe a table or chart. After that, choose the best line of action using the outcomes of your data analysis method.
Data Visualization: In your daily life, data visualizations are relatively common; they frequently take the form of graphs and charts. Alternatively, facts are presented visually to make it simpler for the human brain to comprehend and digest. Data visualization is commonly used to find new patterns and information. You can develop a strategy to obtain valuable information by looking at relationships and contrasting datasets.
Finally, we can say that the data analysis has its own prominence in bringing out substantial support in answering the research problem. Data analysis is a significant and scientific step especially when the researcher is conducting quantitative research. The researcher must understand the research area comprehensively and do the processing, analysis, and finally interpretation with the help of various techniques and tools of analysis depending upon the nature, scope, and aims of the research being conducted.
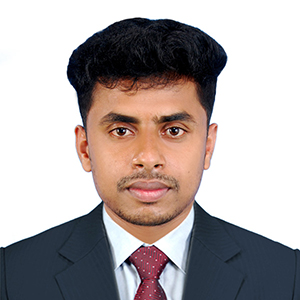
Assistant Teacher at Zinzira Pir Mohammad Pilot School and College