Internet of Things and Big Data Analytics:
In recent years, the emergence of the Internet of Things (IoT) has led to a massive increase in the volume, velocity, and variety of data generated by smart devices. This data, often referred to as Big Data, presents a significant opportunity for organizations to gain insights and make data-driven decisions. However, the sheer volume and complexity of IoT data require new approaches and technologies to extract meaningful insights. In this article, we will explore the connection between the Internet of Things and Big Data Analytics and discuss the opportunities and challenges that arise when working with IoT data.
IoT and Big Data Analytics:
IoT refers to the interconnectivity of devices and sensors embedded in everyday objects, enabling them to collect and exchange data. These devices can range from wearables, home appliances, vehicles, and industrial machinery. The data generated by these devices is often unstructured, heterogeneous, and distributed, making it challenging to analyze and interpret. Moreover, the data generated by IoT devices is often real-time, continuous, and high-volume, which requires new technologies to store, process, and analyze this data.
Big Data Analytics refers to the use of advanced analytical techniques, such as machine learning, data mining, and predictive modeling, to extract insights and knowledge from large and complex datasets. The goal of Big Data Analytics is to transform raw data into actionable insights that can inform business decisions and improve outcomes. The combination of IoT and Big Data Analytics provides organizations with an unprecedented opportunity to gain insights into their operations, customers, and products.
Applications of IoT and Big Data Analytics:
The intersection of the Internet of Things (IoT) and Big Data Analytics presents significant opportunities for organizations across industries to gain insights, make data-driven decisions, and drive innovation. This section will discuss the applications of IoT and Big Data Analytics in various sectors.
- Healthcare: IoT devices can collect real-time data on patient health, providing insights that can inform diagnoses and treatment plans. For example, wearable devices can monitor vital signs, such as heart rate, blood pressure, and oxygen levels, and transmit data to healthcare providers for analysis. Big Data Analytics can process and analyze this data, identifying patterns and trends that can inform diagnoses and treatment plans. Additionally, IoT-enabled telemedicine platforms can connect patients with healthcare providers remotely, expanding access to care and reducing costs.
- Manufacturing: IoT devices can collect data on machine performance, supply chain logistics, and product quality, providing insights that can inform process optimization and improve outcomes. For example, sensors on manufacturing equipment can monitor performance and identify potential issues before they cause downtime or quality issues. Big Data Analytics can process and analyze this data, identifying patterns and trends that can inform process improvements and optimize supply chain logistics.
- Retail: IoT devices can collect data on customer behavior, preferences, and purchase history, providing insights that can inform personalized marketing strategies and enhance customer experiences. For example, beacons in stores can track customer movements and send targeted promotions based on location and past purchase history. Big Data Analytics can process and analyze this data, identifying patterns and trends that can inform product recommendations, pricing strategies, and marketing campaigns.
- Smart Cities: IoT devices can collect data on traffic patterns, energy usage, and environmental conditions, providing insights that can inform infrastructure improvements and enhance the quality of life. For example, sensors on streetlights can monitor traffic patterns and adjust the lighting to improve safety and reduce energy usage. Big Data Analytics can process and analyze this data, identifying patterns and trends that can inform infrastructure improvements and resource allocation.
- Agriculture: IoT devices can collect data on soil moisture, temperature, and nutrient levels, providing insights that can inform precision agriculture practices and optimize crop yields. For example, sensors in fields can monitor soil conditions and irrigation systems, adjusting water and fertilizer levels to optimize crop growth. Big Data Analytics can process and analyze this data, identifying patterns and trends that can inform crop management decisions and improve yields.
- Energy: IoT devices can collect data on energy usage, production, and distribution, providing insights that can inform energy management practices and reduce waste. For example, smart meters can monitor energy usage in homes and businesses, providing real-time data on consumption patterns. Big Data Analytics can process and analyze this data, identifying patterns and trends that can inform energy management practices, including load balancing and demand response.
- Transportation: IoT devices can collect data on vehicle performance, traffic patterns, and logistics, providing insights that can inform route optimization, reduce fuel consumption, and improve safety. For example, sensors on vehicles can monitor fuel consumption and identify potential issues before they cause breakdowns. Big Data Analytics can process and analyze this data, identifying patterns and trends that can inform route optimization, reduce congestion, and improve safety.
Challenges of IoT and Big Data Analytics:
The integration of the Internet of Things (IoT) and Big Data Analytics presents significant opportunities for organizations to gain insights, drive innovation and create value. However, this integration also presents numerous challenges that must be addressed to fully realize the potential benefits. This section will discuss the challenges of IoT and Big Data Analytics in detail.
- Data Management: The sheer volume of data generated by IoT devices can be overwhelming, and managing this data can be a significant challenge. IoT devices generate large amounts of data, and the data must be stored, processed, and analyzed efficiently. Organizations must have the necessary infrastructure, such as cloud computing and storage, to handle the large volumes of data generated by IoT devices. Additionally, the data generated by IoT devices is often unstructured and requires preprocessing to be useful. Data preprocessing involves cleaning, transforming, and aggregating data to prepare it for analysis. This process can be time-consuming and require significant resources.
- Data Security and Privacy: The integration of IoT and Big Data Analytics presents significant security and privacy risks. IoT devices often collect sensitive data, such as personal health information or financial data, which must be protected from unauthorized access. Additionally, IoT devices are often connected to networks, creating potential vulnerabilities that can be exploited by hackers. To mitigate security risks, organizations must implement security measures, such as encryption and access controls, to protect IoT devices and the data they generate. Additionally, organizations must comply with data privacy regulations, such as the General Data Protection Regulation (GDPR) and the California Consumer Privacy Act (CCPA), which regulate the collection, use, and storage of personal data.
- Interoperability: IoT devices are often developed by different manufacturers, and they may use different communication protocols, making it challenging to integrate devices and extract insights from the data they generate. For example, a sensor developed by one manufacturer may use a different communication protocol than a sensor developed by another manufacturer, making it challenging to integrate the data generated by both sensors. To address interoperability challenges, organizations must establish communication standards that allow devices from different manufacturers to communicate with each other. Additionally, organizations must have the necessary tools and expertise to integrate and analyze data from multiple sources.
- Analytics Expertise: To extract insights from the data generated by IoT devices, organizations must have the necessary analytics expertise. Big Data Analytics requires specialized skills, such as data science and machine learning, which may not be available within an organization. Organizations must have the necessary expertise or partner with external service providers to develop and implement effective analytics strategies.
- Cost: The integration of IoT and Big Data Analytics can be expensive, requiring significant investments in hardware, software, and expertise. IoT devises themselves can be costly, and the necessary infrastructure to store and process data can be expensive. Additionally, developing and implementing effective analytics strategies can require significant resources, including expertise and training. To address cost challenges, organizations must carefully evaluate the potential benefits of integrating IoT and Big Data Analytics and develop a clear business case for the investment. Additionally, organizations can explore cost-saving measures, such as partnering with external service providers or using cloud-based solutions.
In conclusion, the intersection of IoT and Big Data Analytics presents significant opportunities for organizations to gain insights and make data-driven decisions. However, to fully realize these opportunities, organizations must address the challenges of working with IoT data, invest in advanced technologies, build a culture of data-driven decision-making, and collaborate with industry, academia, and government. By doing so, organizations can unlock the full potential of IoT and Big Data Analytics and drive innovation, improve outcomes, and enhance customer experiences.
References:
- Gubbi, J., Buyya, R., Marusic, S., & Palaniswami, M. (2013). Internet of Things (IoT): A vision, architectural elements, and future directions. Future Generation Computer Systems, 29(7), 1645-1660.
- Wu, D., Rosen, D. W., & Wang, L. (2016). A framework for smart manufacturing based on the internet of things: reference models, architecture, and implementation. Journal of Manufacturing Systems, 39, 176-194.
- Wang, Y., & Xu, X. (2016). From Big Data to Big Insights: The Impact and Challenges of IoT Technology. IEEE Access, 4, 6144-6153.
- Li, S., Xu, L. D., & Zhao, S. (2015). The internet of things: a survey. Information Systems Frontiers, 17(2), 243-259.
- Raghupathi, W., & Raghupathi, V. (2014). Big data analytics in healthcare: promise and potential. Health Information Science and Systems, 2(1), 3.
- Su, X., Zhou, X., Wei, J., & Zou, J. (2018). Internet of Things (IoT)-enabled healthcare systems: A review of literature and challenges. IEEE/CAA Journal of Automatica Sinica, 5(2), 247-259.
- Zhang, Y., Chen, M., & Hu, J. (2019). Big Data Analytics for IoT-based Smart Homes. IEEE Communications Magazine, 57(6), 44-49.
- Xiong, H., Xiong, Z., & Liu, X. (2016). Big Data Analytics and Applications. CRC Press.
- Iera, A., & Puliafito, A. (2015). Internet of Things in the 5G era: Enablers, architecture, and business models. IEEE Journal on Selected Areas in Communications, 34(3), 510-527.
- Zikopoulos, P., Eaton, C., deRoos, D., Deutsch, T., & Lapis, G. (2011). Understanding Big Data: Analytics for Enterprise Class Hadoop and Streaming Data. McGraw-Hill Osborne Media.
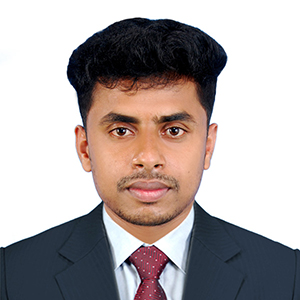
Assistant Teacher at Zinzira Pir Mohammad Pilot School and College