In research, variables are characteristics or properties that can take on different values and are measured or manipulated in order to answer research questions. A research variable can be any measurable factor that can be observed or manipulated, such as age, gender, height, weight, IQ, attitude, behavior, and so on. In the rest of this article, we will discuss different types of research variables.
Definitions of Research Variables:
Here are some definitions of research variables from different sources and perspectives:
“A variable is any characteristic or attribute that can vary or take on different values across individuals, groups, or situations. In research, variables are typically defined in terms of their operational definitions, or how they will be measured or manipulated in the study.” (Maxwell, 2013)
“A research variable is a measurable factor or characteristic that can take on different values or levels. Variables are used in research to test hypotheses, identify relationships between variables, and make predictions about outcomes.” (Babbie, 2017)
“In research, variables are the building blocks of empirical investigations. They are the observable, measurable, and controllable aspects of phenomena that researchers manipulate, observe, or measure to test hypotheses and answer research questions.” (Creswell, 2014)
“A variable is a characteristic or property that can vary or change from one individual, group, or situation to another. In research, variables are typically classified as independent, dependent, or control variables, depending on their relationship to the research question.” (Polit & Beck, 2017)
“A research variable is an attribute or characteristic that can be measured or manipulated in a study. Variables can be either quantitative, such as age or weight, or qualitative, such as attitudes or beliefs. By identifying and measuring variables, researchers can investigate causal relationships and make predictions about behavior.” (Trochim & Donnelly, 2008)
From the above definitions, we can say that research variables are essential components of empirical investigations, as they allow researchers to test hypotheses, identify relationships, and make predictions about outcomes. Understanding the different types of variables and how they are operationalized is crucial for conducting valid and reliable research.
Types of Research Variables:
In research, variables are any measurable or controllable characteristic or property that can take on different values or levels across individuals, groups, or situations. Variables are important in research because they allow researchers to investigate relationships, test hypotheses, and make predictions about behavior. There are three main types of research variables: independent variables, dependent variables, and control variables.
- Independent Variables (IV): An independent variable is a variable that is manipulated or controlled by the researcher in order to observe its effect on the dependent variable. Independent variables are also known as predictor variables because they are used to predict or explain changes in the dependent variable. Independent variables can be either qualitative or quantitative in nature, and they can be measured or manipulated in a variety of ways.
For example, if a researcher wants to study the effect of sleep deprivation on cognitive performance, sleep deprivation would be the independent variable. The researcher would manipulate the amount of sleep that participants receive and then measure their cognitive performance to determine if there is a significant effect. In this case, sleep deprivation is the independent variable, because it is being manipulated by the researcher.
Another example of an independent variable could be age in a study investigating the effect of age on memory performance. The researcher would manipulate the age of the participants, by selecting participants from different age groups, and then measure their memory performance to determine if there is a significant effect.
- Dependent Variables (DV): A dependent variable is a variable that is observed or measured in response to changes in the independent variable. Dependent variables are also known as outcome variables because they are the variables that researchers are interested in predicting or explaining. Dependent variables can be either qualitative or quantitative in nature, and they can be measured in a variety of ways.
For example, in the sleep deprivation study mentioned earlier, cognitive performance would be the dependent variable. The researcher would measure cognitive performance as an outcome of the manipulation of the independent variable (sleep deprivation).
In a study investigating the effect of age on memory performance, memory performance would be the dependent variable. The researcher would measure memory performance as an outcome of the manipulation of the independent variable (age).
- Control Variables: Control variables are variables that are held constant or are controlled in order to minimize their influence on the dependent variable. Control variables are important in research because they allow researchers to isolate the effects of the independent variable on the dependent variable, by minimizing the effects of extraneous variables that could also influence the dependent variable.
For example, in the sleep deprivation study, the researcher might control for the time of day that cognitive performance is measured, to ensure that any changes in cognitive performance are not simply due to circadian rhythms. By holding the time of day constant across participants, the researcher can be more confident that any changes in cognitive performance are due to the manipulation of the independent variable (sleep deprivation).
In a study investigating the effect of age on memory performance, the researcher might control for variables such as education level, socioeconomic status, and health status, because these variables could also influence memory performance. By controlling for these variables, the researcher can be more confident that any changes in memory performance are due to the manipulation of the independent variable (age).
In summary, independent variables, dependent variables, and control variables are important components of research design, because they allow researchers to investigate relationships, test hypotheses, and make predictions about behavior. By manipulating and measuring these variables, researchers can gain insight into the factors that influence human behavior, and develop interventions to improve health, education, and other aspects of human life.
Other Types of Research Variables:
In addition to independent variables, dependent variables, and control variables, there are several other types of variables that are important in research. These include moderating variables, mediating variables, confounding variables, and latent variables.
a. Moderating Variables: A moderating variable is a variable that affects the relationship between the independent variable and the dependent variable. In other words, a moderating variable changes the strength or direction of the relationship between the independent variable and the dependent variable.
For example, in a study investigating the effect of exercise on weight loss, age could be a moderating variable. Age could affect the relationship between exercise and weight loss, such that the relationship might be stronger for younger adults than for older adults. In this case, age would be a moderating variable because it modifies the relationship between the independent variable (exercise) and the dependent variable (weight loss).
b. Mediating Variables: A mediating variable is a variable that explains the relationship between the independent variable and the dependent variable. In other words, a mediating variable provides insight into the underlying mechanisms or processes that link the independent variable to the dependent variable.
For example, in a study investigating the effect of education on income, job skills could be a mediating variable. Job skills might explain how education leads to higher income, because people with more education may acquire better job skills and be more competitive in the job market. In this case, job skills would be a mediating variable because it explains the relationship between the independent variable (education) and the dependent variable (income).
c. Confounding Variables: A confounding variable is a variable that influences both the independent variable and the dependent variable, making it difficult to determine the true relationship between the two variables. Confounding variables are a major concern in research because they can lead to inaccurate or misleading results.
For example, in a study investigating the effect of caffeine on cognitive performance, the time of day could be a confounding variable. If participants consume caffeine in the morning, they may perform better on cognitive tasks than if they consume caffeine in the afternoon, simply because they are more alert in the morning. In this case, time of day would be a confounding variable because it influences both the independent variable (caffeine) and the dependent variable (cognitive performance).
d. Latent Variables: A latent variable is a variable that cannot be directly observed or measured but is inferred from other variables. Latent variables are often used in research when there is no direct way to measure a particular construct or trait.
For example, in a study investigating the effect of personality on job performance, conscientiousness could be a latent variable. Conscientiousness is a trait that cannot be directly observed or measured, but it can be inferred from other behaviors and characteristics, such as being organized, responsible, and reliable. In this case, conscientiousness would be a latent variable because it cannot be directly measured, but it is inferred from other variables.
So, moderating variables, mediating variables, confounding variables, and latent variables are all important types of variables in research. By understanding how these variables operate and interact with other variables in a study, researchers can design more robust and accurate studies that provide insight into the factors that influence human behavior.
At the end of the day, we can say that research variables play a critical role in the research process. By carefully identifying and defining the independent variables, dependent variables, and control variables in a study, researchers can design experiments that allow them to test hypotheses and draw meaningful conclusions about the relationship between variables. Moreover, by recognizing the presence of moderating variables, mediating variables, confounding variables, and latent variables, researchers can refine their studies and account for factors that may influence the results. Overall, a clear understanding of research variables is essential for conducting high-quality research that produces reliable and valid results.
References:
- Cohen, J., Cohen, P., West, S. G., & Aiken, L. S. (2003). Applied multiple regression/correlation analysis for the behavioral sciences. Routledge.
- Field, A. (2013). Discovering statistics using IBM SPSS statistics. Sage publications.
- Hair, J. F., Black, W. C., Babin, B. J., & Anderson, R. E. (2010). Multivariate data analysis: A global perspective. Pearson.
- Jaccard, J., & Turrisi, R. (2003). Interaction effects in multiple regression. Sage publications.
- Johnson, R. A., & Wichern, D. W. (2007). Applied multivariate statistical analysis (Vol. 6). Pearson Prentice Hall.
- Kline, R. B. (2015). Principles and practice of structural equation modeling. Guilford publications.
- MacKinnon, D. P., Lockwood, C. M., & Williams, J. (2004). Confidence limits for the indirect effect: Distribution of the product and resampling methods. Multivariate behavioral research, 39(1), 99-128.
- Pallant, J. (2016). SPSS survival manual. McGraw-Hill Education (UK).
- Preacher, K. J., & Hayes, A. F. (2008). Asymptotic and resampling strategies for assessing and comparing indirect effects in multiple mediator models. Behavior research methods, 40(3), 879-891.
- Raudenbush, S. W., & Bryk, A. S. (2002). Hierarchical linear models: Applications and data analysis methods. Sage.
- Schumacker, R. E., & Lomax, R. G. (2010). A beginner’s guide to structural equation modeling (3rd ed.). Routledge.
- Shrout, P. E., & Bolger, N. (2002). Mediation in experimental and nonexperimental studies: New procedures and recommendations. Psychological Methods, 7(4), 422-445.
- Tabachnick, B. G., & Fidell, L. S. (2013). Using multivariate statistics. Pearson.
- Thompson, B. (2005). Exploratory and confirmatory factor analysis: Understanding concepts and applications. American Psychological Association.
- Van der Linden, W. J., & Hambleton, R. K. (1997). Handbook of modern item response theory. Springer.
- Vittinghoff, E., Glidden, D. V., Shiboski, S. C., & McCulloch, C. E. (2012). Regression methods in biostatistics: linear, logistic, survival, and repeated measures models. Springer Science & Business Media.
- West, S. G., Finch, J. F., & Curran, P. J. (1995). Structural equation models with nonnormal variables: Problems and remedies. In Structural equation modeling: Concepts, issues, and applications (pp. 56-75). Sage.
- Wong, S. S., & Tse, D. K. (2005). Exploring the relationship between environmental uncertainty and self-organization in a dynamic market. Journal of Business Research, 58(6), 821-829.
- Yang, Z., & Peterson, R. T. (2004). Customer perceived value, satisfaction, and loyalty: The role of switching costs. Psychology & Marketing, 21(10), 799-822.
- Zou, G. Y. (2007). Toward using confidence intervals to compare correlations. Psychological Methods, 12(4), 399-413.
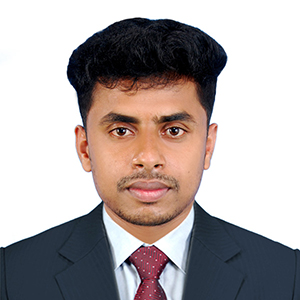
Assistant Teacher at Zinzira Pir Mohammad Pilot School and College