The Reality of AI Research Tools: What They Can and Can’t Do:
Artificial Intelligence (AI) has made significant strides in recent years, offering researchers a range of tools and possibilities. However, it’s crucial to understand the limitations and potential pitfalls of these AI research tools before fully embracing them. In this article, we’ll explore the reality of AI research tools: what they can and can’t do, providing insights into university policies and technological capabilities.
University Policies on AI Research Tools:
As the field of artificial intelligence continues to advance, researchers are presented with an array of AI research tools that have the potential to revolutionize the way they work. However, before researchers eagerly embrace these tools, it is imperative to delve into the intricate web of university policies governing their use. These policies, which can vary significantly from one institution to another, play a pivotal role in shaping the landscape of AI-assisted research within academic settings.
1. The Spectrum of University Policies: At one end of the policy spectrum, some universities adopt an outright ban on the use of AI tools, even for seemingly innocuous tasks such as grammar checking. This approach is notably stringent and may seem extreme, especially considering the ubiquity of AI-driven grammar-checking applications like Grammarly. Such blanket prohibitions may leave researchers frustrated and stifled, particularly when these tools could enhance their work in various ways.
Conversely, on the other end of the spectrum, certain universities are more permissive, allowing students to utilize AI tools under specific conditions. However, these conditions often come with a crucial caveat: students must meticulously cite AI-generated content. This requirement reflects the ethical concerns surrounding AI-generated content and raises pertinent questions about intellectual property ownership.
2. The Conundrum of AI-Generated Content Ownership: One of the most vexing issues associated with AI-generated content revolves around its ownership. When AI algorithms generate content, such as text or data analysis, determining rightful ownership becomes a convoluted affair. Is it the student who initiated the AI’s action? Is it the AI itself, acting as an autonomous creator? Or is it the individuals or organizations that provided the data upon which the AI was trained?
Complicating matters further is the opacity surrounding many AI tools’ data sources. Numerous AI applications do not disclose the origins of their training data, leaving researchers grappling with the challenge of proper citation. This lack of transparency raises concerns about the accuracy, credibility, and traceability of AI-generated outputs.
3. Navigating the Maze of University Policies: Given the myriad complexities surrounding university policies on AI-based support, it is incumbent upon students and researchers to take proactive steps in navigating this maze. Here are some key considerations:
- Awareness and Clarity: Researchers must first familiarize themselves with their institution’s stance on AI tools. This entails thoroughly reviewing university policies, guidelines, and codes of conduct. Moreover, when in doubt, seek clarity from relevant academic authorities or university administrators.
- Supervisor Perspective: Another critical factor to consider is your research supervisor’s perspective on AI tools. Their support or opposition can significantly influence your research journey. Engage in open and transparent discussions with your supervisor to align your research approach with their expectations.
- Ethical Use: Regardless of university policies, researchers should prioritize ethical use of AI tools. This means adhering to guidelines, properly citing AI-generated content, and being transparent about the role of AI in their research.
- Stay Informed: The landscape of AI research is constantly evolving. Policies, technological capabilities, and ethical considerations are subject to change. Researchers should stay informed about updates in this fast-moving space to ensure their work remains in compliance with the latest guidelines.
While AI research tools offer immense potential for researchers, they come with a complex web of university policies and ethical considerations. Researchers must tread carefully, respecting institutional guidelines, understanding the nuances of AI-generated content ownership, and maintaining ethical integrity throughout their research journey. Only through a balanced and informed approach can AI research tools be harnessed effectively within the academic domain.
Navigating the Pitfalls of AI Limitations in Research:
AI tools have undoubtedly ushered in a new era of possibilities for researchers. However, it is vital to approach these tools with a realistic understanding of their limitations and potential pitfalls. While AI holds tremendous promise, it is far from infallible, and researchers must exercise caution when integrating it into their work.
1. Biases in Training Data: One of the most critical limitations of AI, particularly generative AI, is its susceptibility to biases present in its training data. When AI models are trained on data collected from various sources, the biases within that data can seep into the model’s understanding and influence its outputs. For instance, if the training data contains biases related to race, gender, or socio-economic factors, the AI may inadvertently produce biased or unfair content.
These biases can be subtle and insidious, impacting the way AI-generated content is perceived. For researchers, this means that any insights or content produced by AI must be carefully scrutinized for potential bias. AI should be viewed as a tool that can assist in the research process, but researchers must remain vigilant in identifying and addressing bias in the output.
2. Outdated Training Data: Another critical limitation of AI tools, especially those relying on language models, is the potential for outdated training data. AI models, such as natural language processing (NLP) models, are trained on vast datasets collected from the internet. However, the ever-evolving nature of information means that what was accurate and relevant at the time of training may no longer hold true.
Consequently, AI models may produce information that is outdated or no longer applicable. Researchers need to be aware of this limitation and verify the accuracy and currency of any information provided by AI tools. Relying solely on AI-generated content without cross-referencing it with up-to-date sources can lead to inaccuracies in research.
3. Limited Understanding: It’s crucial to recognize that AI, despite its remarkable capabilities, does not truly “understand” in the way humans do. AI, including generative models, operates by recognizing patterns in data and using those patterns to make predictions. In the case of generative AI, it predicts the next word or sequence of words based on the context provided by preceding text.
This lack of genuine understanding means that AI-generated content may sound plausible but is not grounded in true comprehension. Researchers must be cautious when relying on AI-generated explanations, interpretations, or conclusions. These outputs should be considered starting points for further investigation and verification rather than definitive answers.
4. “Hallucinations” in AI Output: A noteworthy concern with generative AI models is the phenomenon known as “hallucinations.” These occur when the AI produces content that seems coherent and accurate but is, in fact, entirely fictional or unsupported by facts. For instance, a generative AI model might generate references in perfect APA format that have no basis in reality.
Researchers must be vigilant in identifying these “hallucinations” in AI-generated content. This underscores the importance of human oversight in the research process. AI should be viewed as a tool that assists researchers in generating ideas, content, or insights, but it should not replace the critical role of human judgment and scrutiny.
In essence, AI research tools should be regarded as junior assistants rather than infallible experts. Researchers must approach AI with a critical eye, ask the right questions, provide clear instructions, and meticulously review the outputs. Moreover, researchers must remain aware of the potential biases, limitations, and “hallucinations” that can arise when using AI. By doing so, researchers can harness the power of AI while ensuring the integrity and reliability of their research endeavors.
AI Research Tools: What They Can Help With:
Despite their limitations, AI research tools can be valuable assets in specific research tasks. We will categorize their potential contributions into four main areas:
1. Finding/Identifying Relevant Literature: An essential component of any research endeavor involves the discovery of pertinent literature that serves as the bedrock of your study. These are the resources that typically find their place in your introduction and literature review chapter. To expedite this crucial phase, there exist several AI research tools that hold the potential to streamline the process.
- Scite Assistant: Scite Assistant stands as a beacon for researchers seeking to navigate the labyrinth of academic literature. With its chat-based interface, it offers answers to research queries, making it easier to identify the most pertinent literature. What sets Scite Assistant apart is its ability to not only provide answers but also furnish a list of references from academic literature to substantiate those answers. This feature ensures that researchers can trace the sources of information back to credible origins, a critical aspect of scholarly research.
- Consensus: Consensus operates akin to a search engine, simplifying the process of finding concise summaries of relevant studies. It offers researchers a streamlined method to explore academic resources related to their research questions. An added convenience is the option to export search results to a CSV file, which can be seamlessly integrated into a researcher’s literature catalogue.
- Research Rabbit: Research Rabbit excels in helping researchers visualize the intricate web of relationships between research papers. It takes the initial set of “starter” articles, often seminal literature in a given field, and creates a visual map of related papers. This map encompasses papers that cite the core articles, as well as those citing the citations themselves. Researchers can also set up alerts to stay informed about new citations—an invaluable feature when research spans an extended period. Visualizing these connections can aid in identifying gaps or overlooked sources.
- Connected Papers: This tool specializes in visualizing the relationships between various papers, offering a unique perspective on academic literature. Researchers can gain insights into how different papers are interconnected and explore the web of knowledge within their research domain. Visual representations can facilitate a deeper understanding of the academic landscape.
While these AI research tools can expedite the initial stages of literature search, they should be considered as facilitators rather than replacements for comprehensive searches. Even with AI assistance, researchers should still undertake traditional literature reviews using platforms like Google Scholar and relevant academic databases to ensure they have identified all relevant sources.
2. Evaluating Source Quality: After establishing a robust foundation of scholarly resources, the subsequent imperative step is the evaluation of source quality. This assessment holds critical significance, as the robustness of your study hinges, at least partially, on the credibility of the literature sources you employ.
- Scite’s “Reference Check”: This tool proves invaluable when assessing the credibility of academic sources. By uploading an article, researchers can obtain a report detailing crucial data points. It reveals how frequently the article has been cited and provides insight into the context of these citations—whether they support or challenge the original article’s claims. While the classifications are AI-generated and not infallible, this functionality offers a quick overview of an article’s reception within the research community.
- Identifying Retracted Sources: Scite’s reference check tool goes a step further by identifying any sources that have been retracted since their publication. This feature serves as an early warning system, helping researchers avoid the inclusion of discredited content in their work. However, due diligence is essential to verify the accuracy of these claims.
3. Building a Literature Catalogue: As previously discussed, the construction of a comprehensive literature catalogue holds paramount importance in the synthesis and comprehension of scholarly materials. To facilitate this crucial task, there exist AI-based research tools that can expedite the process effectively.
- Elicit: Elicit streamlines the process of building a comprehensive literature catalogue. Researchers can upload their collection of journal articles, and the tool will extract key pieces of information, allowing them to create a structured spreadsheet. Researchers can define columns for essential details such as region, population, study type, and more. While researchers must verify the accuracy of the extracted information, Elicit serves as a time-saving starting point.
- Petal: Petal introduces a chat-based interface for interacting with PDFs, particularly useful when seeking specific information within articles. Researchers can engage with their PDFs and extract pertinent details. However, as with any AI tool, it is imperative to cross-check the extracted data for accuracy.
It is crucial to remember that while AI tools like Elicit and Petal can assist in compiling a literature catalogue, they should not substitute in-depth engagement with the literature. Researchers must maintain a profound understanding of the articles they intend to cite, ensuring their ability to provide a critical synthesis in their literature review.
4. Improving Writing: The fourth potential application of AI to enhance your research project revolves around the writing aspect. However, it’s imperative to meticulously adhere to your university’s policies to avoid any complications. Therefore, ensure that you have a comprehensive understanding of what is permissible and what is not within your academic institution’s guidelines.
- Grammarly: Grammarly is a versatile tool that aids researchers in improving the quality of their writing. Beyond basic grammar and spelling checks, Grammarly offers suggestions for sentence structure, word choice, and tone. It functions as a comprehensive writing assistant, helping researchers refine their written work.
- ChatGPT: ChatGPT, while primarily known for its conversational abilities, can be a valuable resource for researchers. It can assist in articulating ideas more clearly and concisely. Researchers can paste their text into ChatGPT and use prompts like “rewrite this paragraph for clarity” to refine their prose. Additionally, ChatGPT excels in generating analogies and examples, enhancing the explanatory power of research.
It is essential to exercise caution when using AI for writing tasks. Researchers must ensure that their use of AI aligns with their university’s policies, particularly when it comes to generative AI that can produce content autonomously.
AI research tools offer an array of capabilities to researchers, significantly streamlining various aspects of the research process. However, researchers should approach these tools with a discerning eye, utilizing them as aids rather than substitutes and ensuring compliance with university policies and ethical standards. When wielded effectively, AI research tools can empower researchers to conduct more efficient and impactful investigations.
In conclusion, AI research tools offer valuable support to researchers but come with limitations and ethical considerations. It’s vital for students and researchers to understand their university’s policies, exercise caution when relying on AI-generated content, and remain informed about the evolving landscape of AI in research. While AI tools can expedite certain research tasks, they should be viewed as supplements to, not replacements for, human-driven research efforts.
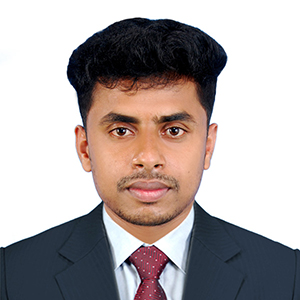
Former Student at Rajshahi University