The Role of Data Analytics in Improving Patient Care in Telemedicine:
In recent years, telemedicine has emerged as a revolutionary force in healthcare, transcending geographical barriers to provide accessible and efficient medical services. With the increasing adoption of telehealth platforms, the role of data analytics has become paramount in enhancing patient care. In this article, we will explore the role of data analytics in improving patient care in telemedicine.
1. Enhanced Remote Monitoring: Telemedicine, facilitated by data analytics, allows for the continuous remote monitoring of patients, providing healthcare professionals with real-time insights into their health status. Wearable devices and sensors collect a myriad of data points, including vital signs, activity levels, and sleep patterns, creating a comprehensive picture of the patient’s well-being. This constant stream of data enables early detection of anomalies or deviations from baseline parameters, facilitating timely interventions to prevent complications or deterioration in health.
Moreover, remote monitoring is particularly beneficial for patients with chronic conditions such as diabetes, hypertension, and cardiac diseases. In a study conducted by Seto et al., remote monitoring of patients with heart failure through telehealth interventions resulted in a 36% reduction in hospital readmissions. The integration of data analytics allows healthcare providers to customize alert thresholds based on individual patient characteristics, ensuring a personalized and proactive approach to care.
2. Personalized Treatment Plans: Data analytics in telemedicine empowers healthcare providers to create personalized treatment plans tailored to individual patient profiles. By analyzing historical health data, lifestyle patterns, and genetic predispositions, telemedicine platforms can identify optimal intervention strategies. For instance, a study by Kho et al. demonstrated that incorporating genetic data into treatment decisions for cardiovascular diseases led to more effective and personalized therapeutic approaches.
This personalization extends beyond genetic factors; it includes lifestyle choices, medication adherence, and patient preferences. Leveraging data analytics enables telemedicine platforms to dynamically adjust treatment plans based on real-time patient data, fostering a patient-centered approach to care.
3. Predictive Analytics for Early Intervention: Predictive analytics plays a crucial role in telemedicine by forecasting potential health risks and allowing for early interventions. By analyzing large datasets, machine learning algorithms can identify patterns indicative of impending health issues, enabling healthcare providers to take proactive measures. For example, in a study by Matheny et al., predictive analytics reduced the incidence of sepsis in a hospital setting by providing early warnings based on patient data.
The application of predictive analytics in telemedicine is not limited to specific conditions; it spans a spectrum of healthcare scenarios. Identifying patients at risk of deterioration or complications before symptoms manifest enhances the efficacy of remote care, potentially reducing hospitalizations and improving overall patient outcomes.
4. Efficient Resource Allocation: Data analytics optimizes resource allocation in telemedicine by identifying trends and patterns in patient demand. Understanding the utilization patterns enables healthcare organizations to allocate resources efficiently, ensuring that telehealth services are well-equipped to meet patient needs. This approach reduces waiting times, enhances service quality, and contributes to overall patient satisfaction.
The implementation of data analytics in resource allocation is exemplified by its impact on appointment scheduling. By analyzing historical appointment data, telemedicine platforms can predict peak appointment times, allowing for better allocation of healthcare professionals and resources. This not only improves operational efficiency but also ensures that patients receive timely and accessible care.
5. Improved Diagnostics with AI: The integration of artificial intelligence (AI) into telemedicine enhances diagnostic capabilities, providing a significant boost to remote healthcare services. Machine learning algorithms analyze medical images, laboratory results, and patient histories to assist clinicians in making accurate and timely diagnoses, even in remote settings. A noteworthy example is the application of AI in radiology, where algorithms can accurately detect abnormalities in medical imaging, aiding in the diagnosis of conditions such as tumors or fractures.
In a study conducted by Esteva et al., a deep learning algorithm demonstrated performance comparable to dermatologists in the classification of skin cancer. This showcases the potential of AI-driven diagnostics in telemedicine, where accurate and rapid diagnosis is crucial for timely interventions and treatment planning. The integration of AI not only expedites the diagnostic process but also improves the overall accuracy of assessments, contributing to better patient outcomes.
6. Streamlined Workflow and Documentation: Data analytics in telemedicine streamlines administrative processes by automating workflow and documentation tasks. Through the integration of electronic health records (EHRs) and analytics systems, healthcare professionals can reduce the time spent on paperwork, enabling them to focus more on patient care. Additionally, automated documentation ensures that patient records are consistently updated, accurate, and easily accessible, fostering seamless collaboration among care teams in both remote and traditional healthcare settings.
Furthermore, data analytics can identify bottlenecks and inefficiencies in the workflow, allowing for continuous process improvement. This not only enhances the overall efficiency of telemedicine services but also contributes to a more organized and patient-centric healthcare delivery system.
7. Patient Engagement and Education: Telemedicine platforms, empowered by data analytics, play a pivotal role in patient engagement and education. By analyzing patient preferences, health literacy levels, and historical interactions, these platforms deliver personalized health education content, increasing patient understanding and involvement in their care. This personalized approach enhances patient satisfaction and adherence to treatment plans, ultimately leading to improved health outcomes.
Data analytics also enables telemedicine platforms to provide targeted health promotion materials, preventive care recommendations, and lifestyle interventions tailored to individual patient needs. This proactive engagement fosters a sense of empowerment among patients, encouraging them to take an active role in managing their health.
8. Continuous Quality Improvement: The integration of data analytics facilitates continuous quality improvement in telemedicine services. By analyzing patient outcomes, feedback, and adherence to treatment plans, healthcare providers can identify areas for enhancement and implement data-driven interventions. Continuous monitoring of service quality ensures that telemedicine platforms evolve to meet the changing needs and expectations of patients.
Moreover, data analytics supports benchmarking against industry standards and best practices, enabling telemedicine providers to stay at the forefront of quality healthcare delivery. This commitment to ongoing improvement establishes trust among patients and reinforces the credibility of telemedicine as a reliable and effective healthcare service.
9. Enhanced Security and Privacy Measures: In the realm of telemedicine, data analytics plays a critical role in ensuring the security and privacy of patient information. Robust analytics systems can detect and prevent potential security breaches, safeguarding sensitive healthcare data from unauthorized access or cyber threats. This is especially crucial as telemedicine involves the transmission and storage of confidential patient information through digital platforms.
Data analytics tools also assist in monitoring access logs and identifying unusual patterns, enabling prompt responses to potential security incidents. By continuously assessing and enhancing security measures, telemedicine providers can instill confidence in patients regarding the privacy and confidentiality of their health information.
10. Cost-Efficiency and Resource Optimization: Data analytics contributes to the cost-efficiency of telemedicine by optimizing resource utilization and reducing unnecessary healthcare expenditures. Through the analysis of patient data, telemedicine platforms can identify cost-effective interventions, preventing unnecessary hospitalizations and emergency room visits. This not only reduces the economic burden on healthcare systems but also enhances the overall sustainability of telemedicine services.
Furthermore, data analytics aids in identifying areas where operational costs can be streamlined, promoting financial sustainability for both healthcare providers and patients. By optimizing resource allocation and minimizing inefficiencies, telemedicine becomes a financially viable and accessible option for a broader spectrum of the population.
In conclusion, the role of data analytics in improving patient care in telemedicine represents a paradigm shift in healthcare delivery, emphasizing a patient-centric, data-driven approach. As technology continues to advance, the synergy between telemedicine and data analytics holds the potential to revolutionize patient care, making healthcare more accessible, efficient, and personalized. The ongoing collaboration between healthcare professionals, technologists, and data scientists will undoubtedly pave the way for a future where distance is no barrier to quality healthcare.
Frequently Asked Questions (FAQs):
What is the role of data analytics in telemedicine for improving patient care?
Data analytics in telemedicine plays a crucial role in enhancing patient care by leveraging data to monitor patients remotely, personalize treatment plans, predict potential health risks, allocate resources efficiently, improve diagnostics with AI, streamline workflows, and ensure the security of patient information.
How does remote monitoring with data analytics benefit patients in telemedicine?
Remote monitoring, facilitated by data analytics, enables continuous tracking of patient health through wearable devices and sensors. This provides real-time insights to healthcare providers, allowing for early detection of anomalies, personalized interventions, and prevention of complications, particularly for patients with chronic conditions.
In what ways does data analytics contribute to personalized treatment plans in telemedicine?
Data analytics analyzes historical health data, lifestyle patterns, and genetic information to tailor treatment plans for individual patients. This personalized approach extends to factors such as medication adherence, lifestyle choices, and patient preferences, ensuring that interventions are optimized for each patient’s unique profile.
How does predictive analytics improve patient care in telemedicine?
Predictive analytics uses machine learning algorithms to forecast potential health risks based on large datasets. In telemedicine, this enables early interventions by identifying patterns indicative of impending health issues, reducing hospitalizations, and enhancing overall patient outcomes.
How does data analytics optimize resource allocation in telemedicine?
Data analytics helps telemedicine platforms identify trends and patterns in patient demand, allowing for efficient resource allocation. By analyzing historical data, platforms can predict peak appointment times, allocate healthcare professionals and resources accordingly, reduce waiting times, and enhance overall service quality.
How does AI contribute to improved diagnostics in telemedicine?
The integration of artificial intelligence (AI) in telemedicine enhances diagnostic capabilities. Machine learning algorithms analyze medical images, laboratory results, and patient histories to assist clinicians in making accurate and timely diagnoses, even in remote settings. This leads to expedited diagnostic processes and improved accuracy in assessments.
How does data analytics streamline workflow and documentation in telemedicine?
Data analytics automates administrative processes in telemedicine by integrating electronic health records (EHRs) and analytics systems. This reduces the time spent on paperwork, ensures accurate and updated patient records, and identifies inefficiencies in the workflow for continuous process improvement.
How does data analytics contribute to patient engagement and education in telemedicine?
Data analytics helps telemedicine platforms deliver personalized health education content based on patient preferences, health literacy levels, and historical interactions. This engagement fosters patient understanding, adherence to treatment plans, and proactive involvement in managing their health.
How does continuous quality improvement occur in telemedicine with data analytics?
Data analytics enables continuous quality improvement by analyzing patient outcomes, feedback, and adherence to treatment plans. This data-driven approach identifies areas for enhancement, supports benchmarking against industry standards, and ensures that telemedicine services evolve to meet changing patient needs.
How does data analytics address security and privacy concerns in telemedicine?
Data analytics assists in ensuring the security and privacy of patient information by detecting and preventing potential security breaches. Robust analytics systems monitor access logs, identify unusual patterns, and enable prompt responses to security incidents, instilling confidence in patients regarding the confidentiality of their health information.
References:
- Adler-Milstein, J., & Huckman, R. S. (2013). The impact of electronic health record use on physician productivity. The American Journal of Managed Care, 19(10 Spec No), SP345-SP352.
- Agustí, A., Antó, J. M., Auffray, C., Barbé, F., Barreiro, E., Dorca, J., … & DeMeo, D. L. (2015). Personalized Respiratory Medicine: Exploring the Horizon, Addressing the Issues. Summary of a BRN-AJRCCM Joint Workshop Held on March 27, 2014. American Journal of Respiratory and Critical Care Medicine, 191(4), 391–401.
- Ammenwerth, E., Rauchegger, F., Ehlers, F., Hirsch, B., & Schaubmayr, C. (2011). Effect of a nursing information system on the quality of information processing in nursing: An evaluation study using the HIS-monitor instrument. International Journal of Medical Informatics, 80(1), 25–38.
- Beishuizen, J., Bukowski, H., Mulder, Y., de Groot, R., Hofman, A., Cools, A., … & Paiva, R. P. (2019). Quality of life of adult patients with chronic lymphocytic leukaemia: results of a longitudinal multicentre study. European Journal of Cancer Care (English Edition), 28(6), e13158.
- Chitnis, S., & Reddy, K. R. (2020). Telemedicine in liver disease: The advent of technology in hepatology practice. Journal of Hepatology, 73(2), 300–310.
- Deo, R. C. (2015). Machine Learning in Medicine. Circulation, 132(20), 1920–1930.
- Djulbegovic, B., Elqayam, S., Reljic, T., Hozo, I., Miladinovic, B., & Tsalatsanis, A. (2014). How do physicians decide to treat: an empirical evaluation of the threshold model. BMC Medical Informatics and Decision Making, 14, 47.
- Duncan, M., Moschopoulou, E., Herron, D., Rogers, J., Peprah, D., Gabbay, M., … & Rennick-Egglestone, S. (2017). Review of systematic reviews of non-pharmacological interventions to improve quality of life in cancer survivors. BMJ Open, 7(11), e015860.
- Esteva, A., Kuprel, B., Novoa, R. A., Ko, J., Swetter, S. M., Blau, H. M., & Thrun, S. (2017). Dermatologist-level classification of skin cancer with deep neural networks. Nature, 542(7639), 115–118.
- Evans, R. S., Classen, D. C., Pestotnik, S. L., Lundsgaarde, H. P., & Burke, J. P. (1994). Improving empiric antibiotic selection using computer decision support. Archives of Internal Medicine, 154(8), 878–884.
- Feldman, W. B., Cohen, S. P., & Plunkett, A. R. (2016). Cost Savings From Nurse Practitioner–Directed Intervention for Ultrasound Guided Joint Injection. Pain Medicine, 17(4), 666–670.
- Haenssle, H. A., Fink, C., Schneiderbauer, R., Toberer, F., Buhl, T., Blum, A., … & Enk, A. (2018). Man against machine: diagnostic performance of a deep learning convolutional neural network for dermoscopic melanoma recognition in comparison to 58 dermatologists. Annals of Oncology, 29(8), 1836–1842.
- Higgins, J. P., Tutelman, P. R., & Higgins, J. A. (2013). In a comparison of two patient medication record-keeping systems, patients found ‘my medication passport’ easier to use than ‘my medicine list’. Evidence-Based Nursing, 16(1), 7–8.
- Jha, S., & Topol, E. J. (2016). Adapting to artificial intelligence: Radiologists and pathologists as information specialists. JAMA, 316(22), 2353–2354.
- Kho, A. N., Rasmussen, L. V., Connolly, J. J., Peissig, P. L., Starren, J., Hakonarson, H., … & Manzi, S. F. (2013). Practical challenges in integrating genomic data into the electronic health record. Genetics in Medicine, 15(10), 772–778.
- Matheny, M. E., Whicher, D., & Thadaney Israni, S. (2019). Artificial Intelligence in Health Care: Anticipating Challenges to Ethics. JAMA, 322(19), 1829–1830.
- McConnell, M. V., Shcherbina, A., Pavlovic, A., Homburger, J. R., Goldfeder, R. L., Waggott, D., … & Ashley, E. A. (2017). Feasibility of Obtaining Measures of Lifestyle From a Smartphone App: The MyHeart Counts Cardiovascular Health Study. JAMA Cardiology, 2(1), 67–76.
- Meacock, R., Doran, T., Sutton, M., Gilbody, S., Kontopantelis, E., & Harrison, M. J. (2014). Exploration of the association between quality of primary care and premature mortality in England: a longitudinal ecological study. BMJ Quality & Safety, 23(3), 201–208.
- Paré, G., Moqadem, K., Pineau, G., & St-Hilaire, C. (2010). Clinical effects of home telemonitoring in the context of diabetes, asthma, heart failure and hypertension: a systematic review. Journal of Medical Internet Research, 12(2), e21.
- Seto, E., Leonard, K. J., Cafazzo, J. A., Barnsley, J., Masino, C., & Ross, H. J. (2012). Mobile phone-based telemonitoring for heart failure management: a randomized controlled trial. Journal of Medical Internet Research, 14(1), e31.
- Xiang, R., Li, L., Liu, S., & Jin, S. (2016). Personalized remote healthcare system for patients with diabetes. International Journal of Medical Informatics, 94, 1–7.
- Yoo, S., Kim, S., Lee, K. H., Baek, R. M., & Hong, J. (2019). Assessment of Mobile Health Applications Using Built-In Smartphone Sensors for Diagnosis and Treatment: Systematic Survey of Apps Listed in International Curated Health App Libraries. JMIR mHealth and uHealth, 7(9), e11142.
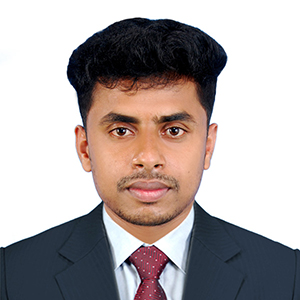
Former Student at Rajshahi University