Role of Inferential Statistics in Research:
In the vast realm of research, drawing meaningful conclusions from data is an essential task. This is where inferential statistics come into play, providing researchers with powerful tools to make sense of their findings. Inferential statistics allow us to move beyond numbers and charts, enabling the formulation of solid hypotheses and informed decision-making. In this article, we will explore the pivotal role of inferential statistics in research.
1. Drawing Conclusions: Inferential statistics play a fundamental role in drawing conclusions that extend beyond the specific data collected in a research study. When researchers conduct experiments or surveys, they typically work with a sample of the population they are interested in. Inferential statistics allow them to make valid inferences about the entire population based on the observations made in the sample.
For example, consider a political poll where a sample of 1,000 voters is surveyed to gauge public opinion about a particular candidate. Inferential statistics can help draw conclusions about the entire voting population. If 60% of the sample supports the candidate, inferential statistics can provide a level of confidence that the candidate’s actual level of support in the entire population falls within a certain range, such as 55% to 65%. This range is known as a confidence interval, and it helps to quantify the uncertainty associated with the conclusion.
2. Hypothesis Testing: Hypothesis testing is a critical step in the scientific method. Researchers formulate hypotheses about relationships or effects they expect to find in their data. Inferential statistics are used to rigorously test these hypotheses and determine whether the observed results are statistically significant.
Let’s say a pharmaceutical company develops a new drug and wants to test whether it’s more effective than an existing medication. They set up an experiment with a control group (using the existing drug) and a treatment group (using the new drug). After collecting and analyzing the data, inferential statistics can help determine if the differences observed between the two groups are significant or if they could have occurred by random chance.
The significance level, often denoted by “p-value,” is a common output of hypothesis testing. If the p-value is less than a predetermined threshold (e.g., 0.05), researchers can conclude that their results are statistically significant, indicating a real difference between the treatments.
3. Generalization: In research, the goal is not merely to describe the characteristics of a sample but to generalize findings to a larger population. Inferential statistics enable this generalization by allowing researchers to make reliable statements about a population based on a sample.
Imagine a sociologist studying the attitudes of a specific demographic group towards climate change. By collecting data from a sample of this group, they can make informed generalizations about the entire population of that demographic. Inferential statistics help ensure that the findings apply beyond the sample, making research more relevant and impactful.
4. Estimation: Estimation is a critical aspect of research, especially when it’s impractical to measure an entire population. Inferential statistics enable researchers to estimate population parameters, such as the mean, proportion, or variance, with a degree of confidence.
For example, an environmental scientist researching air pollution may collect air quality measurements from a limited number of monitoring stations across a city. Inferential statistics can be used to estimate the average pollution level for the entire city based on the data from these stations. The estimate is accompanied by a confidence interval, indicating the range within which the true population parameter is likely to fall.
5. Identifying Relationships: Researchers often seek to identify and quantify relationships or associations between variables. Inferential statistics not only help detect these relationships but also assess their statistical significance. This is crucial for determining whether observed connections are likely to hold true in the broader population or if they could have occurred by random chance.
For instance, a psychologist studying the relationship between hours of sleep and cognitive performance in a sample of students may use inferential statistics to determine if there is a statistically significant correlation between the two variables. If such a relationship is confirmed, it provides a basis for making inferences about the general population of students and the importance of sleep on cognitive performance.
6. Prediction: Inferential statistics play a pivotal role in predictive modeling. Researchers often aim to make informed predictions about future outcomes based on historical data and relationships identified in their samples. By using inferential statistics, they can develop models that forecast future events, trends, or behaviors with a certain level of confidence.
For example, in the field of finance, stock market analysts use historical stock price data and various economic indicators to build predictive models. These models employ inferential statistics to make forecasts about the future performance of stocks and investment opportunities, assisting investors in their decision-making processes.
7. Comparing Groups: Inferential statistics are essential when researchers need to compare different groups or conditions to determine if there are statistically significant differences between them. This process is crucial in various fields, from clinical trials in medicine to A/B testing in marketing.
For instance, a pharmaceutical company conducting a clinical trial on a new drug may use inferential statistics to assess whether the treatment group (receiving the new drug) exhibits significantly better outcomes compared to the control group (receiving a placebo or existing medication). The results obtained through inferential statistics help determine the drug’s effectiveness.
8. Decision-Making: Inferential statistics are not limited to research; they extend to practical decision-making across numerous domains. Whether it’s in business, healthcare, or social sciences, inferential statistics provide a robust foundation for data-driven decisions.
Consider a scenario where a retail company wants to optimize its inventory management. By analyzing sales data and using inferential statistics, the company can make informed decisions about how much of each product to stock, when to restock, and when to offer discounts. This data-driven decision-making can lead to reduced costs and increased profits.
9. Quality Control: In manufacturing and process industries, maintaining product quality is paramount. Inferential statistics are instrumental in quality control, ensuring that products meet specific standards and specifications. Instead of inspecting every item produced, manufacturers use inferential statistics to sample products and assess their quality.
For example, an automobile manufacturer might use inferential statistics to sample a subset of cars from a production line and assess their safety and performance. If the sample meets quality standards, the manufacturer can confidently conclude that the entire production meets those standards, saving time and resources while ensuring product quality.
10. Policy Development: In the realm of public policy, evidence-based decisions are essential to address societal challenges effectively. Researchers and policymakers rely on inferential statistics to develop policies grounded in data and empirical research.
For instance, when creating environmental policies, government agencies may use inferential statistics to assess the impact of various regulations on air and water quality. By analyzing data from representative samples, they can make evidence-based policy recommendations that are more likely to achieve the desired environmental outcomes while considering economic and social implications. Inferential statistics ensure that policies are founded on sound scientific findings and contribute to the betterment of society.
In conclusion, inferential statistics, while a complex field in itself, is a vital aspect of research across various domains. Its role in drawing conclusions, hypothesis testing, generalization, estimation, identifying relationships, prediction, comparing groups, decision-making, quality control, and policy development cannot be overstated. Without inferential statistics, the foundation of evidence-based decision-making and scientific progress would be shaky at best. Researchers, policymakers, and businesses alike depend on these tools to unlock the hidden insights in data and drive innovation and progress. In essence, inferential statistics forms the bridge that connects the research conducted on a small scale to the wider world, making it an indispensable part of the scientific process.
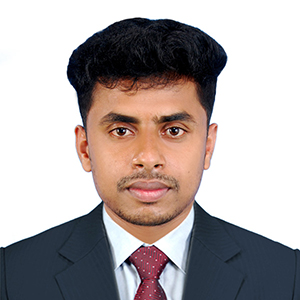
Assistant Teacher at Zinzira Pir Mohammad Pilot School and College