The Big 3 Qualitative Coding Approaches: Inductive, Deductive, and Abductive
Qualitative research often relies on coding as a systematic means of categorizing and interpreting data. The three primary coding approaches—inductive, deductive, and abductive—guide researchers in analyzing qualitative data effectively (Miles, Huberman, & Saldaña, 2014). Each approach serves a distinct purpose and is applied based on the research objective, theoretical framework, and nature of the data collected. This article explores the big 3 qualitative coding approaches: inductive, deductive, and abductive.
What is Qualitative Coding?
Qualitative coding is the analytical process of identifying, categorizing, and interpreting patterns within qualitative data. It is a fundamental step in qualitative research, allowing researchers to systematically organize and make sense of textual, visual, or audio data (Saldaña, 2021). Coding provides a structured way to navigate unstructured data by assigning labels—known as codes—to meaningful segments of text, helping researchers uncover underlying themes and insights.
From a grounded theory perspective, qualitative coding is a means of theory generation. Charmaz (2014) describes coding as a way to construct theory from data, emphasizing an iterative and emergent process where researchers remain open to new themes arising from the data rather than imposing pre-existing concepts. In grounded theory research, coding is conducted in multiple phases, such as initial open coding, focused coding, and theoretical coding, to refine and develop conceptual categories.
From a thematic analysis perspective, Braun and Clarke (2006) view coding as an essential step in identifying themes within qualitative datasets. They highlight that coding can be either data-driven (inductive) or theory-driven (deductive), depending on whether the researcher aims to generate new insights or apply existing theoretical frameworks. Thematic analysis involves a flexible yet rigorous process, where coding is used to identify patterns that contribute to a coherent understanding of the research topic.
From a phenomenological perspective, coding is a way to capture lived experiences and the essence of human perceptions. Van Manen (1990) argues that qualitative coding in phenomenology should remain deeply interpretative, ensuring that codes preserve the rich, subjective meaning of participants’ experiences. Instead of breaking down data into rigid categories, phenomenological coding seeks to maintain the holistic essence of a phenomenon by emphasizing descriptive and reflective codes.
From a practical research methodology perspective, Miles, Huberman, and Saldaña (2014) emphasize the pragmatic role of coding in data reduction and pattern recognition. They argue that coding is not merely a mechanical task but a critical process that requires constant reflection and refinement. Their approach integrates coding with qualitative data visualization techniques, such as matrices and network diagrams, to enhance analytical rigor.
Simply put, qualitative coding is the process of categorizing and labeling textual data to lay the foundation for identifying themes, patterns, and ultimately, insights. It is the first step toward qualitative data analysis.
In practical terms, coding involves meticulously reading through a dataset—such as interview transcripts, field notes, or documents—and assigning ‘codes’ to various excerpts from the text. These codes can be words, phrases, or short summaries that capture the essence of each data segment.
Example of Qualitative Coding: Imagine you have an interview transcript where a participant discusses their experience with a specific online learning platform. A segment of the transcript might read: “I found online classes challenging because I struggled with time management and staying motivated.” When coding this transcript, you might assign codes like “Time Management Challenges” and “Motivation Issues” to this specific passage. In other words, you’d be labeling and categorizing snippets of text as you work your way through the transcript.
The exact pieces of text you decide to label and which specific codes you use will depend on the coding structure you adopt, as well as your research aims and research questions.
The Big 3 Qualitative Coding Approaches:
Having established a clear understanding of qualitative coding, we can now delve into the three primary approaches to coding: inductive, deductive, and hybrid (also referred to as abductive) coding. Let us examine each of these methods:
1. Inductive Coding: Inductive coding is a bottom-up approach to qualitative data analysis where codes and themes emerge directly from the data rather than being predetermined by existing theories or frameworks (Thomas, 2006). This method allows researchers to remain open to new patterns and insights, making it particularly useful in exploratory studies and grounded theory research (Charmaz, 2014).
Characteristics of Inductive Coding:
- Data-Driven Approach – Codes are developed organically based on patterns observed in the data, rather than being imposed by prior knowledge.
- Exploratory Nature – Often used in studies where little is known about the research topic, allowing unexpected themes to surface.
- Iterative Process – Involves multiple rounds of coding and refinement, ensuring that themes accurately represent the data.
- Flexibility – Unlike deductive coding, which applies a fixed set of codes, inductive coding allows the coding scheme to evolve as analysis progresses.
Process of Inductive Coding:
- Familiarization with Data – The researcher reads and rereads transcripts, notes, or documents to gain an overall sense of the content.
- Generating Initial Codes – Segments of text that convey meaningful information are labeled with descriptive codes.
- Clustering Similar Codes – Codes that share common meanings are grouped into broader categories.
- Developing Themes – Emerging categories are refined into overarching themes that represent the central ideas in the data.
- Review and Refinement – The themes are reviewed and adjusted to ensure they align with the overall dataset and research objectives.
- Final Interpretation – The identified themes are analyzed in relation to the research questions, forming the basis for study conclusions.
Example of Inductive Coding: Consider a study examining students’ experiences with remote learning. An interview participant states:
“At first, online learning was confusing. I had trouble keeping up with assignments, and I missed the face-to-face interaction with classmates.”
Using an inductive approach, the researcher might assign the following initial codes:
- Confusion with Online Learning – Reflecting the initial struggle to adapt.
- Challenges with Assignment Management – Indicating difficulty in keeping up with coursework.
- Lack of Social Interaction – Highlighting the absence of peer engagement.
As more data is analyzed, similar codes are grouped, and overarching themes such as “Adaptation Difficulties” and “Social Isolation in Online Learning” may emerge.
Advantages of Inductive Coding:
- Unbiased and Data-Driven – Minimizes researcher bias by allowing themes to emerge naturally from the data.
- Captures Rich Detail – Ensures nuanced and context-specific insights, essential for qualitative research.
- Encourages Theory Development – Often used in grounded theory research to construct new theories from empirical findings.
Challenges of Inductive Coding:
- Time-Consuming – Requires extensive reading and iterative refinement of codes.
- Subjectivity in Interpretation – Relies on researcher judgment, which may introduce bias.
- Potential for Overgeneralization – Without a clear theoretical framework, researchers must ensure themes accurately represent diverse perspectives in the data.
2. Deductive Coding: Deductive coding is a top-down approach to qualitative data analysis where predefined codes are applied to the data based on existing theories, frameworks, or research questions (Crabtree & Miller, 1999). Unlike inductive coding, which allows themes to emerge from the data, deductive coding starts with a structured set of codes that guide the analysis process.
Characteristics of Deductive Coding:
- Theory-Driven Approach – Coding is based on established theories, prior research, or conceptual frameworks.
- Structured and Systematic – Researchers use a predetermined set of codes, ensuring consistency across the dataset.
- Focused Analysis – Emphasizes specific themes or constructs relevant to the research objectives.
- Efficient and Time-Saving – Since codes are predefined, data analysis can be more streamlined and faster than inductive coding.
Process of Deductive Coding:
- Developing a Codebook – Researchers create a structured list of predefined codes based on theoretical constructs or prior research.
- Applying Codes to Data – The predefined codes are systematically applied to relevant text segments.
- Checking for Additional Codes – Although the approach is structured, researchers may refine the codebook if unexpected insights emerge.
- Grouping and Analyzing Codes – Coded data is organized into themes, ensuring alignment with the research framework.
- Interpreting Findings – The coded themes are analyzed in relation to the study’s research questions and theoretical perspectives.
Example of Deductive Coding: Consider a study investigating student engagement in online learning using Self-Determination Theory (Deci & Ryan, 1985), which includes three main constructs: Autonomy, Competence, and Relatedness. If a participant states:
“I struggle to stay motivated in online classes because I don’t feel connected to my peers or instructors.”
Using deductive coding, the researcher might apply the following predefined codes:
- Relatedness – Lack of connection with peers and instructors.
- Motivation Issues – Struggle to maintain engagement in online learning.
Since the codes are drawn from Self-Determination Theory, the analysis remains structured and aligned with the research framework.
Advantages of Deductive Coding:
- Ensures Consistency – Using predefined codes helps maintain a structured and systematic approach.
- Efficient and Focused – Speeds up data analysis by applying a predetermined framework.
- Aligns with Established Theories – Strengthens the connection between findings and existing literature.
Challenges of Deductive Coding:
- May Overlook Emerging Themes – Predefined codes can limit the discovery of new or unexpected patterns.
- Potential for Confirmation Bias – Researchers may focus only on themes that fit the theoretical framework.
- Less Flexibility – Compared to inductive coding, it offers fewer opportunities for codes to evolve based on data.
3. Hybrid (Abductive) Coding: Hybrid coding, also known as abductive coding, is a combined approach that integrates both inductive and deductive coding strategies. It allows researchers to apply predefined codes based on existing theories while remaining open to new themes emerging from the data (Fereday & Muir-Cochrane, 2006). This approach balances structure and flexibility, making it particularly useful for studies that aim to validate existing knowledge while also exploring novel insights.
Characteristics of Hybrid (Abductive) Coding:
- Combines Deductive and Inductive Approaches – Uses an initial coding framework while allowing for new, data-driven codes to emerge.
- Iterative and Reflexive – Researchers continuously refine codes, adjusting between predefined and emergent categories.
- Enhances Theoretical and Empirical Rigor – Maintains alignment with theoretical constructs while capturing unexpected themes.
- Flexible but Structured – Provides a balance between efficiency and adaptability in qualitative analysis.
Process of Hybrid (Abductive) Coding:
- Developing an Initial Codebook – A predefined set of codes is created based on existing theories or research questions.
- Applying Predefined Codes – The researcher begins by coding data using the established framework.
- Identifying Emerging Codes – As analysis progresses, new patterns or concepts that do not fit the initial codes are noted.
- Refining the Codebook – Both predefined and emergent codes are revised, ensuring they accurately represent the data.
- Final Analysis and Thematic Development – The combined set of codes is used to generate overarching themes and insights.
Example of Hybrid (Abductive) Coding: Imagine a study examining employee well-being in remote work environments using the Job Demands-Resources (JD-R) model (Bakker & Demerouti, 2007). The model suggests that job demands (e.g., workload, isolation) and job resources (e.g., support, autonomy) influence well-being. If a participant states:
“Working from home has been great for my work-life balance, but I sometimes feel disconnected from my colleagues.”
Using hybrid coding:
- Predefined codes from JD-R model:
- Job Resources – Work-Life Balance (based on positive remote work experiences)
- Job Demands – Social Isolation (lack of connection with colleagues)
- Emergent code:
- Flexibility as a Double-Edged Sword (capturing both the benefits and challenges of flexible work arrangements)
This approach ensures that existing theoretical categories are applied while allowing for novel themes to emerge.
Advantages of Hybrid (Abductive) Coding:
- Balances Structure and Discovery – Ensures theoretical alignment while allowing room for new insights.
- Enhances Analytical Depth – Captures both expected and unexpected themes in qualitative data.
- Increases Credibility and Rigor – Helps validate findings by integrating both deductive and inductive perspectives.
Challenges of Hybrid (Abductive) Coding:
- Requires Skilled Interpretation – Researchers must carefully balance predefined codes with emergent themes.
- Time-Intensive – Iterative refinement of codes requires substantial effort and reflexivity.
- Risk of Theoretical Bias – Over-reliance on existing theories may limit the emergence of genuinely new insights.
How to Choose the Right Coding Approach:
Selecting the appropriate qualitative coding approach depends on several factors, including research objectives, theoretical orientation, data characteristics, and the level of structure required in analysis. Below are key considerations for choosing between inductive, deductive, and hybrid (abductive) coding approaches.
i. Research Goals and Questions:
- Inductive Coding: Best suited for exploratory research where little prior knowledge exists, allowing themes to emerge organically from the data (Thomas, 2006).
- Deductive Coding: Ideal for studies that aim to test or apply existing theories, using predefined codes for structured analysis (Crabtree & Miller, 1999).
- Hybrid Coding: Useful for research that both validates existing theories and remains open to discovering new insights (Fereday & Muir-Cochrane, 2006).
ii. Theoretical Framework:
- If your research is grounded in an established theory, deductive coding ensures alignment with theoretical constructs.
- If you aim to develop a new theory from data, inductive coding is more appropriate.
- Hybrid coding is effective when balancing existing theoretical concepts with emerging data patterns.
iii. Nature of the Data:
- Inductive Coding: Suitable when working with open-ended interviews, focus groups, or ethnographic observations where themes are not predetermined.
- Deductive Coding: Works well for structured surveys, policy analysis, or studies using existing conceptual frameworks.
- Hybrid Coding: Beneficial for complex datasets that require both structured organization and flexibility in theme development.
iv. Time and Resource Constraints:
- Inductive Coding: Requires significant time and effort, as codes evolve iteratively.
- Deductive Coding: More efficient when working with large datasets or when time constraints exist, as it follows a predefined structure.
- Hybrid Coding: May require more analytical effort since it combines both structured coding and emergent theme identification.
v. Researcher’s Experience and Bias Considerations:
- Inductive Coding: Minimizes initial researcher bias by allowing the data to drive coding.
- Deductive Coding: Risk of confirmation bias, as predefined codes may limit the discovery of unexpected insights (Patton, 2015).
- Hybrid Coding: Helps mitigate bias by allowing researchers to refine existing codes while integrating new findings.
Decision-Making Summary Table:
Factor | Inductive Coding | Deductive Coding | Hybrid Coding |
Exploratory Research | ✅ Best choice | ❌ Not ideal | ✅ Suitable |
Theory-Driven Research | ❌ Less relevant | ✅ Best choice | ✅ Suitable |
Flexibility | ✅ High | ❌ Low | ✅ Moderate |
Time Efficiency | ❌ Time-consuming | ✅ More efficient | ❌ Moderately time-consuming |
Bias Control | ✅ Low bias | ❌ Higher risk of bias | ✅ Balanced approach |
The choice of qualitative coding approach depends on research needs, theoretical alignment, and available resources. Inductive coding is best for exploratory research, deductive coding is ideal for theory-driven studies, and hybrid coding offers a balanced approach for integrating structured frameworks with emerging insights. By carefully considering these factors, researchers can ensure that their coding method aligns with the goals of their qualitative analysis.
It is apparent that understanding the distinctions between inductive, deductive, and abductive coding is essential for qualitative researchers aiming to analyze data effectively. While inductive coding fosters data-driven discovery, deductive coding offers structure and hypothesis testing. Abductive coding, meanwhile, provides a balanced approach that integrates empirical findings with theoretical insights. Selecting the appropriate coding method depends on the research purpose, dataset characteristics, and theoretical framework.
Frequently Asked Questions (FAQs):
What is qualitative coding?
Qualitative coding is the process of categorizing and labeling textual data to identify themes, patterns, and insights in qualitative research.
What is the difference between inductive, deductive, and abductive coding?
- Inductive coding: Codes emerge from the data without a predefined framework.
- Deductive coding: Codes are predefined based on existing theories or literature.
- Abductive (Hybrid) coding: Combines both inductive and deductive approaches, allowing for theory-driven analysis while incorporating emerging themes.
When should I use inductive coding?
Use inductive coding when conducting exploratory research with little prior knowledge of the subject or when you want to let themes naturally emerge from the data.
When is deductive coding most useful?
Deductive coding is best suited for research based on existing theories, frameworks, or specific research questions that require structured data analysis.
What are the advantages of hybrid (abductive) coding?
Hybrid coding balances structure and flexibility by applying a theoretical framework while allowing for new themes to emerge. It is useful for studies aiming to validate existing theories while also exploring novel insights.
How do I choose the right coding approach for my study?
Consider your research goals, theoretical framework, nature of the data, time constraints, and the need for flexibility versus structure. If you need to explore new themes, use inductive coding; if you are testing theories, use deductive coding; if you want a balanced approach, use hybrid coding.
Can I switch coding approaches during my research?
Yes, researchers often refine their coding approach as they analyze data. For example, they may start with deductive coding and later incorporate inductive elements if new themes emerge.
What tools can I use for qualitative coding?
Popular qualitative analysis tools include NVivo, ATLAS.ti, MAXQDA, and Dedoose, which help manage and organize coded data efficiently.
How do I ensure reliability in qualitative coding?
To enhance reliability, use clear coding definitions, involve multiple coders for consistency, conduct inter-coder reliability checks, and document coding decisions throughout the analysis process.
Is one coding approach superior to the others?
No, each approach has its strengths and is best suited for specific research contexts. The choice depends on your study’s objectives and the level of theoretical guidance required.
References:
- Bakker, A. B., & Demerouti, E. (2007). The Job Demands-Resources model: State of the art. Journal of Managerial Psychology, 22(3), 309-328. https://doi.org/10.1108/02683940710733115
- Braun, V., & Clarke, V. (2006). Using thematic analysis in psychology. Qualitative Research in Psychology, 3(2), 77–101. https://doi.org/10.1191/1478088706qp063oa
- Charmaz, K. (2014). Grounded Theory in Global Perspective: Reviews by International Researchers. Qualitative Inquiry, 20(9), 1074-1084. https://doi.org/10.1177/1077800414545235
- Crabtree, B. F., & Miller, W. L. (1999). Doing qualitative research (2nd ed.). SAGE Publications.
- Deci, E. L., & Ryan, R. M. (1985). Intrinsic motivation and self-determination in human behavior. Springer. https://doi.org/10.1007/978-1-4899-2271-7
- Fereday, J., & Muir-Cochrane, E. (2006). Demonstrating rigor using thematic analysis: A hybrid approach of inductive and deductive coding and theme development. International Journal of Qualitative Methods, 5(1), 80-92. https://doi.org/10.1177/160940690600500107
- Miles, M.B., Huberman, A.M. and Saldana, J. (2014) Qualitative Data Analysis: A Methods Sourcebook. SAGE Publications.
- Patton, M. Q. (2015). Qualitative research & evaluation methods: Integrating theory and practice (4th ed.). SAGE Publications.
- Saldana, J. (2021). The Coding Manual for Qualitative Researchers. Thousand Oaks, CA: SAGE Publications Limited.
- Thomas, D. R. (2006). A general inductive approach for analyzing qualitative evaluation data. American Journal of Evaluation, 27(2), 237-246. https://doi.org/10.1177/1098214005283748
- van Manen, M. (1990) Researching lived experience: Human science for an action sensitive pedagogy. State University of New York Press, Albany.
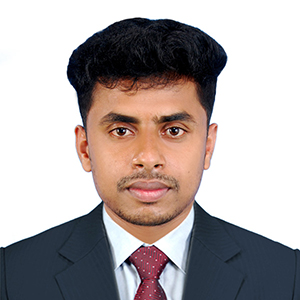
Assistant Teacher at Zinzira Pir Mohammad Pilot School and College