Essential Steps to Become a Data Mining Team Leader:
Data mining, the process of extracting valuable insights from large and complex datasets, has become a crucial component in decision-making across various industries. As the demand for skilled professionals in this field continues to rise, the role of a data mining team leader has emerged as a key position responsible for overseeing projects, managing teams, and effectively communicating results to stakeholders. In the rest of this article, we will explore some of the essential steps to become a data mining team leader.
Step 1: Assess Your Skills: The foundation of a successful career in data mining begins with a thorough self-assessment of your current skill set. A competent data mining professional must possess a comprehensive understanding of key domains, including data management, statistics and machine learning, programming, and data visualization.
- Data Management: A data mining team leader needs to be adept at handling large and complex datasets. This involves understanding data storage, retrieval, and manipulation techniques. Proficiency in database management systems and data warehousing is essential.
- Statistics and Machine Learning: Strong statistical knowledge forms the bedrock for making informed decisions based on data patterns. Additionally, a grasp of machine learning algorithms is vital for predictive modeling and uncovering hidden insights within datasets.
- Programming: Competence in programming languages such as SQL, Python, and R is crucial. These languages are the tools through which data mining professionals interact with and manipulate data, making them essential skills for effective team leadership.
- Data Visualization: The ability to communicate complex findings visually is paramount. A data mining team leader should be familiar with visualization tools like Tableau, enabling them to present insights in a clear and understandable manner to both technical and non-technical stakeholders.
To evaluate your skills, consider leveraging online tests, courses, and certifications. Seeking feedback from peers and mentors provides valuable external perspectives, guiding your focus on areas that require improvement. This initial self-assessment lays the groundwork for targeted professional development.
Step 2: Gain Practical Experience: Beyond theoretical knowledge, practical experience is indispensable for excelling in the dynamic realm of data mining. The second step involves actively engaging in data mining projects to showcase your ability to solve real-world problems using data.
- On-the-Job Experience: Look for opportunities within your current role to contribute to data mining tasks. This could involve collaborating with colleagues in other departments, identifying areas where data insights can drive decision-making, and actively participating in relevant projects.
- Online Platforms and Competitions: Joining online platforms, competitions, or communities dedicated to data science and mining provides exposure to diverse problem-solving scenarios. Platforms like Kaggle offer a competitive yet collaborative environment where you can apply your skills, learn from others, and gain recognition.
- Documentation and Portfolio Building: Document your data mining projects meticulously, detailing the challenges faced, methodologies employed, and results achieved. Building a comprehensive portfolio or maintaining a blog not only demonstrates your skills to potential employers but also serves as a personal record of your growth and achievements.
Gaining practical experience not only fortifies your skill set but also positions you as a candidate with a proven track record, making you more appealing to prospective employers.
Step 3: Develop Leadership Skills: Transitioning from a data mining professional to a team leader demands the cultivation of effective leadership skills. A data mining team leader must be proficient in defining goals, planning and executing processes, delegating tasks, monitoring performance, and effectively communicating findings.
- Goal Definition: Clearly defining data mining goals and objectives is the first step. This involves understanding the broader business objectives and aligning the team’s efforts to contribute meaningfully towards achieving them.
- Planning and Execution: Efficiently planning and executing the data mining process requires a strategic approach. This involves breaking down larger tasks into manageable steps, allocating resources effectively, and ensuring timelines are met.
- Delegation and Coordination: Delegating tasks to team members based on their strengths and expertise is a critical leadership skill. Effective coordination ensures that each team member’s contribution aligns seamlessly, leading to a cohesive and successful project outcome.
- Performance Monitoring and Evaluation: Regularly monitoring and evaluating team performance is essential for identifying areas of improvement and acknowledging achievements. Implementing feedback loops allows for continuous refinement and optimization of the data mining process.
- Communication and Presentation: The ability to communicate and present findings is paramount. A data mining team leader must convey complex technical insights in a comprehensible manner to both technical and non-technical stakeholders. This involves leveraging data visualization tools and crafting compelling narratives.
Investing in leadership development through courses, books, or participation in leadership programs is essential. Additionally, cultivating soft skills such as motivation, coaching, and mentorship contributes to fostering a collaborative and innovative team culture.
Step 4: Expand Your Network: Building a robust professional network is instrumental in advancing your career in data mining. Networking not only exposes you to industry trends and best practices but also facilitates connections with other professionals, experts, and leaders in the field. Here’s how you can effectively expand your network:
- Attend Events and Conferences: Actively participate in events, conferences, and workshops related to data mining. These gatherings provide opportunities to meet professionals from diverse backgrounds, exchange ideas, and stay updated on the latest developments in the field. Engaging in discussions during these events can broaden your perspective and introduce you to potential mentors or collaborators.
- Join Online Groups and Forums: The digital age has made it easier than ever to connect with professionals worldwide. Join online groups, forums, or platforms dedicated to data mining. Websites like LinkedIn, Stack Overflow, and specialized data science communities offer spaces for networking, sharing insights, and seeking advice from experienced professionals.
- Build an Online Presence: Establishing a strong online presence is crucial in the digital era. Share your data mining projects, articles, or insights on social media platforms, blogs, or podcasts. Creating and curating valuable content not only showcases your expertise but also attracts like-minded professionals to your network.
- Seek Mentors and Advisors: Actively seek mentors or advisors who have experience in data mining leadership roles. A mentor can provide guidance, share their experiences, and offer valuable insights that can significantly contribute to your professional development. Establishing a mentorship relationship can be a rewarding aspect of your networking efforts.
- Engage in Knowledge Exchange: Networking is a two-way street. Actively engage in knowledge exchange by offering your expertise and insights to others in the field. This reciprocal approach fosters meaningful connections and positions you as a valuable contributor to the data mining community.
Step 5: Apply for Leadership Roles: With a refined skill set, practical experience, and an expanded network, the final step involves actively seeking and applying for data mining leadership roles. Here’s a strategic approach to applying for these positions:
- Research the Market and Industry: Conduct thorough research on the current state of the data mining market and industry trends. Identify companies, organizations, or sectors that actively seek data mining leaders. Understanding the demand for these roles enables you to tailor your approach and target the most relevant opportunities.
- Tailor Your Resume and Portfolio: Customize your resume, cover letter, and portfolio to highlight your data mining skills, practical experience, and leadership abilities. Clearly articulate your achievements and contributions in previous roles, emphasizing how your skills align with the requirements of a leadership position.
- Prepare for Data Mining Interviews: Data mining leadership roles often involve rigorous interview processes. Prepare for these interviews by anticipating questions related to technical proficiency, analytical skills, communication abilities, and your strategic vision for leading a data mining team. Use concrete examples from your experience to showcase your capabilities.
- Demonstrate Your Unique Value Proposition: During the application process and interviews, emphasize your unique value proposition. Highlight how your approach to data mining, leadership style, and problem-solving skills set you apart from other candidates. Articulate a clear vision for how you would lead a team to success in achieving data mining goals.
- Stay Persistent and Adaptable: The job search process may require persistence and adaptability. Be open to opportunities that align with your career goals, even if they differ slightly from your initial expectations. Maintain flexibility and a willingness to learn and grow in new leadership roles.
In conclusion, becoming a data mining team leader is a rewarding journey that requires a combination of technical expertise, practical experience, leadership skills, and a strong professional network. By following the steps outlined in this comprehensive guide, you can position yourself as a qualified and capable leader in the dynamic and evolving field of data mining. Remember, continuous learning and adaptation are key to staying at the forefront of this rapidly advancing field.
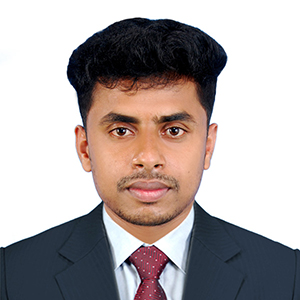
Assistant Teacher at Zinzira Pir Mohammad Pilot School and College